Enterprise AI Agents in 2025: Lessons from Box, Glean & Cohere on Automating Workflows
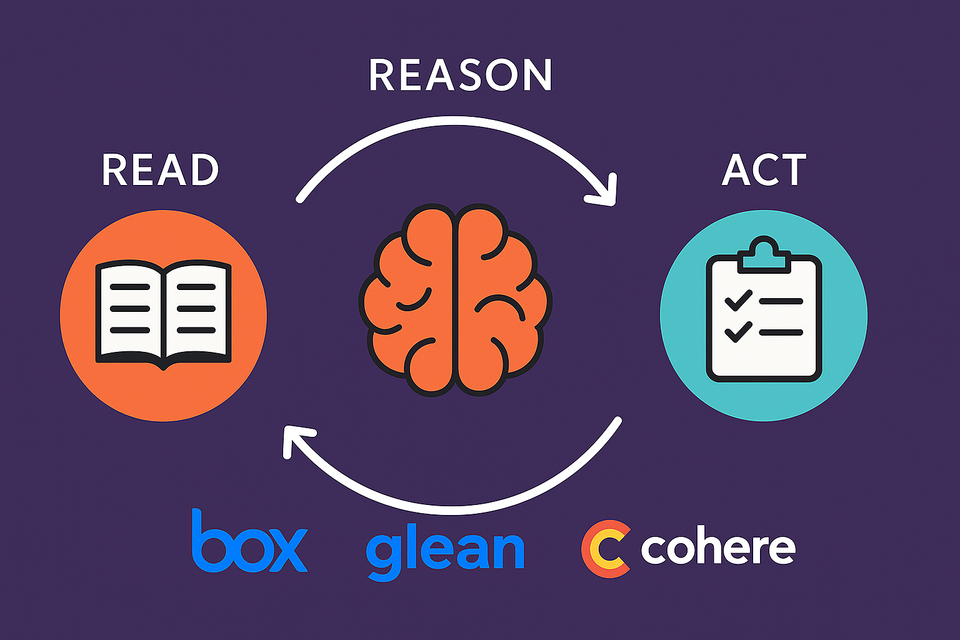
AI’s splashiest tricks—photo‑perfect headshots, celebrity voice‑overs, instant Tik‑Tok scripts—aren’t where the real money is hiding. As Box CEO Aaron Levie quips, we’re “over‑indexed on the flashiest parts of AI in the consumer world and under‑indexed on the things that will make hundreds of billions of dollars in B2B.”
Boards are feeling the heat: enterprise buyers now walk into meetings saying, “We know this is going to change everything… every employee needs it.” Their end‑game isn’t another chatbot—it’s a quiet productivity revolution where each of us commands a team of AI agents, multiplying our output ten‑fold and reshaping how entire industries run.
This week’s briefing dives beneath the consumer hype to unpack that massive, slower‑moving wave—and why the next Fortune 500 winners will be the companies that master it first.
Insights from 3 Enterprise AI CEOs
This newsletter distills insights from recent podcast interviews with three CEOs at the forefront of enterprise AI: Aidan Gómez (Cohere), Arvind Jain (Glean), and Aaron Levie (Box). Each leads a company reshaping how large organizations deploy AI to automate workflows and enhance productivity.
Cohere, specializing in large language models tailored for enterprise use, has achieved an annualized revenue of $35 million as of March 2024, reflecting its growing impact in the AI sector.
Glean, focusing on AI-powered workplace search and automation, reached $100 million in annual recurring revenue in its last fiscal year, underscoring its rapid adoption in the enterprise space.
Box, a pioneer in cloud content management, reported $279.5 million in revenue for Q4 FY2025, marking a 6% year-over-year increase, and continues to integrate AI to expand its enterprise offerings. Together, their perspectives offer a comprehensive view of the current landscape and future trajectory of AI in enterprise settings.
1. Why Enterprise AI ≠ Consumer AI
Consumer AI dazzles by showing what a model can do; enterprise AI wins only when it can be trusted to do it every day, inside the messy, regulated back office. Aaron Levie captures the gap bluntly: consumer models can already answer “What’s the score?” or “Tell me about WW II,” but the unsolved—and lucrative—20 percent is, “Book the flight and file the expense automatically.”
That difference has three practical consequences:
- Risk & Governance trump novelty. Bank CISOs who once dragged their feet on cloud adoption now sprint toward AI—yet they still demand airtight security boundaries, role‑based data access, and audit trails before a single token is generated. “You can’t just turn on an AI thing that looks at all my data,” Levie reminds us, “because it will reveal secrets employees were over‑provisioned for five years ago.”
- Data chaos is part of the job description. Glean’s Arvind Jain warns founders against blaming poor results on “bad internal data” and walking away. “You can never ‘clean’ enterprise data first,” he says. The winning products instead learn to sift obsolete slides, half‑written specs, and noisy support tickets in real time, “figuring out what’s the right information for any task” before the LLM reasons over it.
- Models alone don’t cut it—workflows and guarantees matter. Cohere’s Aidan Gomez calls the foundation model merely “the heart of the company.” To sell into Fortune 100s you must also ship reliability SLAs, deployment inside a private VPC, and a playbook that short‑circuits the endless proof‑of‑concept loop.
Consumer wave | Enterprise wave | |
---|---|---|
Trigger | ChatGPT “wow” demo | Board‑level mandate (“It’s not if but how,” Levie) |
Biggest blocker | Model quality | Trust, security, compliance (Cohere deploys in‑VPC or on‑prem to reach “the most sensitive data”) |
Adoption speed | Bottom‑up virality | Change‑management & architecture decisions (data access, permissions) |
Key success metric | Daily active users | Time‑to‑value for the workflow |
Put differently, consumer AI is a magic trick; enterprise AI is a mission‑critical supply chain. And in that supply chain, governance, retrieval quality, and outcome‑based guarantees are the differentiators—not a one‑point bump on MMLU.
2. Where AI Agents Already Earn Their Keep
“Agents” became Silicon Valley’s new buzzword the moment ChatGPT unveiled custom GPTs, but in the boardrooms Cohere, Glean and Box sell to, an agent isn’t a shiny chatbot—it’s a production micro‑service that reads, reasons and acts on a workflow so a human doesn’t have to. All three CEOs report that, despite the hype, genuine product‑market‑fit has emerged in only a few, very specific veins:
2.1 Document & Data Extraction—quietly huge
Box’s first shipping agent ingests a contract or invoice, identifies the critical fields, drops them into a database, and triggers the follow‑up task (approval, payment, alert).
“Customers keep throwing use‑cases at us—we can’t keep up with demand.” — Aaron Levie
The value prop is tangible: replace paralegal or accounts‑payable hours with seconds of GPU time, while eliminating copy‑paste errors. Levie predicts entire niche software categories (e.g., contract‑lifecycle management) will balloon from single‑digit‑billion to $20 B+ markets when priced as outcome‑automation rather than seats.
2.2 Outbound Sales & SDR Automation
Levie calls lead‑gen the “maybe only clear PMF in agents right now,” citing portfolio company 11x and rivals that personalise first‑touch email campaigns at human‑like quality and machine scale. An agent here isn’t composing generic outreach; it mines CRM, news feeds, and LinkedIn for context, proposes tailored value props, and iterates until quota is hit.
2.3 Coding & Internal IT Copilots
Cursor, Replit, Windsurf and similar tools sit somewhere between autocomplete and full agent. They shine when the task is non‑strategic but time‑consuming: refactor an in‑house admin panel, generate migrations, or convert a legacy script.
“You’d have paid a contract dev firm six months to build the thing—now an agent does it overnight.” — Levie
2.4 Knowledge‑Ops Agents—Glean’s internal experiment
Glean turned its own roadmap‑planning ritual into an agent: it listens to every recorded customer call, extracts feature requests, clusters them into themes, then spits out a spreadsheet and narrative summary for PMs.
“It replaced weeks of anecdotal interviews with one query—and the team trusts the result because it heard every voice, not just the loud ones.” — Arvind Jain
2.5 Vertical RAG Assistants—insurance, healthcare, manufacturing
Cohere slots its models behind domain‑specific retrieval engines:
- Insurance actuaries cut RFP research time so dramatically that they now win more bids.
- Healthcare systems pass decades‑long patient records through an agent that returns a targeted clinical briefing “in under a second.”
“The average doctor cannot read twenty years of notes before a 15‑minute appointment, but the model can.” — Aidan Gómez
Pattern to copy
Every successful deployment follows the same skeleton:
- Read – Securely retrieve the minimal, relevant slice of enterprise data.
- Reason – Chain‑of‑thought or tool‑calling to reach a decision.
- Act – Post the update, file the ticket, send the email, or trigger the next micro‑workflow—no human intermediary.
If your candidate workflow doesn’t need all three stages, you may only need search or summarisation, not a full agent.
3. Build, Buy, or Fine‑Tune? A Practical Decision Framework
The consumer world loves leaderboard bragging rights; the enterprise world worries about TCO, latency, and lock‑in. All three CEOs articulate a remarkably similar rubric:
“Start with the cheapest, simplest path—fine‑tune.
Work backwards only if the business case justifies it.” — Aidan Gómez, Cohere
3.1 Cohere’s “Capability Pyramid”
- Base: General‑purpose models (open‑source or commercial) solve 80 % of generic Q&A, text generation, summarisation.
- Middle: Vertical SaaS tools (e.g., legal brief writers, medical coders) wrap those models in domain data and UX.
- Peak: Enterprise‑specific pre‑training or heavy post‑training, justified only when you sit on “hundreds of billions of proprietary tokens and a problem no one will sell to you.” Building here is a strategic moat, not a science project.
3.2 Levie’s Open‑Weights Litmus Test
Box benchmarked Google’s open‑weights Gemma against the closed Gemini‑Pro for document data‑extraction; Gemma scored “within two percentage points.”
“At that point the choice isn’t accuracy; it’s whether you want the scaffolding managed for you.” — Levie
Take‑away: When open models close the quality gap, your moat must live in workflow integration, security guarantees, and proprietary feedback loops, not raw model IP.
3.3 Glean’s Economics of Custom Models
Arvind Jain frames model choice as unit economics rather than technology vanity:
- Early‑stage startups with low traffic should “be happy to overpay for GPT‑4—you barely have users; optimise cost later.”
- High‑volume endpoints (support classification, real‑time autocomplete) may merit distilled specialist models to keep margins healthy.
- Training from scratch makes sense only when no available model handles the task (rare) or when inference costs swamp the business line (e‑commerce search, ad scoring).
3.4 A Decision Table You Can Re‑use
Trigger question | Default path | Escalate to… | CEO sound‑bite |
---|---|---|---|
Off‑the‑shelf model hits ≥95 % task accuracy and token spend ≤15 % of gross margin? | Prompt engineering + small fine‑tunes | — | “Why spend millions if a prompt and 1K examples work?” — Gómez |
Accuracy gap remains but traffic is modest (<10 RPS)? | Fine‑tune closed or open model with LoRA / SFT | — | “Cheapest lever first.” — Gómez |
Traffic large, latency/cost critical? | Distil or post‑train a smaller expert model | — | “Cost matters only when it threatens margins.” — Jain |
Own unique, large corpus (>100 B tokens) and need frontier‑level performance? | — | Partner on partial pre‑training or self‑host open‑weights | “A $5 M continuation run can match GPT‑4 in your domain.” — Gómez |
Data must never leave VPC / on‑prem? | Self‑host open‑weights, or vendor with on‑prem deploy (Cohere) | — | “Security is the deal‑breaker in finance & health.” — Gómez |
Key mindset shift: treat model choice like any other buy‑vs‑build calculus—ROI, margin impact, strategic differentiation—not a hack‑day flex. Your end‑user values the workflow that closes a ticket or drafts a contract, not the number of parameters under the hood.
4. Data & Architecture Playbook
How to wire “Read → Reason → Act” without painting yourself into a corner.
4.1 Start with Retrieval, not the LLM
Enterprise data is noisy, stale and permissioned. Glean’s Arvind Jain argues that good products assume that reality and make retrieval a first‑class component, not an after‑thought wrapped around the model.
“Blaming poor answers on bad data misses the point. The winner learns to sift obsolete slides, half‑written specs and noisy tickets in real time, deciding what to feed the model for each task.”
In practice that means:
- ingest everything (docs, tickets, call recordings),
- store in a vector or hybrid index with row‑level ACLs,
- inject only the minimal, permission‑checked slice into the prompt.
The result isn’t just privacy compliance; it reduces context size, latency and cost.
4.2 Modular Layers, Swappable Brains
Aaron Levie’s engineering mantra inside Box is “lock what won’t change; abstract what will.” Some parts of the stack are stable (auth, persistence). Others—the model provider, vector search, RAG strategy—“change every three minutes” and therefore live behind clean interfaces so they can be hot‑swapped.
A minimal modern stack therefore has:
- Source connectors → continuously stream SaaS, DB and object‑store content.
- Semantic index → vector + metadata store that enforces ACLs.
- Policy & guardrail layer → governs prompt injection defence, PII scrubbing, rate limits.
- Model router → chooses between open‑weights, commercial, or distilled experts based on task, cost and privacy needs.
- Agent orchestrator → manages multi‑step plans, tool calls and retries.
Change in one layer (e.g., swap Gemma for Claude‑3) should require only a config file edit, not a full rewrite.
4.3 Guardrails Are a Feature, not an Add‑on
Cohere’s enterprise wins often hinge on its ability to run inside a customer’s VPC or fully on‑prem so that regulated data never crosses the public internet. But Gómez notes that security is only half the trust equation; deterministic behaviour is the other half.
“All language models are quite sensitive to how you present data. Folks over‑estimate them, implement RAG badly, watch the POC fail, and blame the model. Structured APIs and predictable formatting cut those failures dramatically.”
A robust policy layer therefore does more than block profanity:
- canonicalises prompts,
- enforces schema on tool outputs,
- retries or falls back to safer models under uncertainty,
- logs every decision for audit.
4.4 Build Feedback Loops from Day 1
Levie reminds us that the moat migrates from the base model to the embedding‑level network effects created by customer data and interactions. Each extraction or classification enriches the index, nudging the agent’s next answer a little closer to perfect. Over time that “opaque data source” becomes the stickiness that UI screens once provided.
Instrument your pipelines: capture retrieval hits, chosen tools, human overrides. Feed that telemetry back into re‑ranking, fine‑tuning or guardrail rules. Observability isn’t a luxury; it is how the system learns and how you prove ROI to a skeptical CFO.
Conclusion
By combining retrieval‑first thinking with modular boundaries, policy guardrails and continuous feedback, you create an architecture that absorbs the next model breakthrough instead of being broken by it.
5. Pricing & Go‑to‑Market — From Seat Licences to “Tasks Automated”
The SaaS boom rode a simple formula: price per user, bill monthly. AI agents break that logic. They aren’t users, they’re labour substitutes—or, as Aaron Levie puts it, “a form of elastic head‑count.” When software starts doing the work a human once did, the value metric must migrate from “active seats” to “units of work completed.”
5.1 Service‑as‑Software: capturing the labour budget
Levie projects that agent spend will dwarf the licence fees of the pre‑AI tools they augment:
“Contract‑management software is a couple‑billion‑dollar category. Legal services are hundreds of billions. Let AI capture 10 % of that and suddenly you’re running a $20 B market.”
In other words, pricing should key off business outcomes—contracts processed, invoices reconciled, leads qualified—because that’s where the displaced budget lives.
5.2 Usage‑based, but not token‑based
Raw token billing is easy to meter, but it exposes vendors to rapid price compression as open‑source and hyperscalers drive per‑token costs toward zero. Cohere offers a different lever:
“You can double the customer’s intelligence threshold today—no retrain needed—by simply letting the model reason longer at inference. That unlocks a metered ‘smartness tier’ the buyer can pay for.” — Aidan Gómez
Instead of charging for compute volume, Cohere frames the bill around compute depth (basic, enhanced‑reasoning, premium‑reasoning), aligning revenue with the incremental value the customer perceives.
5.3 Designing SKUs around “Jobs to be Done”
Glean renamed its licensing axis from “search seats” to “workflows automated.” A sales‑ops manager might license the “Pipeline Hygiene Agent” (deduplicates leads nightly) while a PM pays for the “Roadmap Insights Agent.” Internally, Glean tracks these SKUs against clear time‑saved metrics so customers can convert automation into ROI in the next Quarterly Business Review.
“If the agent removes a task an associate used to spend ten hours a week on, that’s the metric we sell and renew on.” — Arvind Jain
5.4 Beware the Deflationary Spiral
Competitive pressure will still squeeze margins: open‑weights models approach parity (Gemma within two points of Gemini‑Pro on Box’s evals), and rivals pitch lower‑cost automation every quarter. Levie’s antidote is network effects: each customer’s proprietary data and feedback loop improves the product just for them, raising the cost of switching and letting the vendor defend premium pricing.
5.5 Practical checklist for PMs & founders
- Define the atomic unit of value. Is it documents parsed, tickets closed, or revenue‑qualified leads?
- Meter what the CFO already budgets for. If your agent replaces $50/hour labour, align price to a discount on that, not GPU seconds.
- Offer smartness tiers, not just consumption tiers. Let customers pay more for deeper reasoning or stricter guarantees.
- Instrument outcome telemetry from day 1. Without usage-to-value dashboards, your champion can’t defend renewal.
- Layer in data‑network effects. Productised feedback loops (fine‑tuning, re‑ranking, custom rules) justify sticking power—and premium ARPU.
6. Org & Skill Shifts — Building Teams for a Model‑Every‑Quarter World
“The foundation underneath you is changing every three minutes.
Don’t get attached—be ready to throw away what you built two months ago.”
— Aaron Levie
6.1 From Product Cycles to Continuous Prototyping
Traditional enterprise release trains—requirements, six‑month build, GA—cannot absorb monthly model upgrades or shifting open‑source baselines. Cohere’s Gómez asks his teams to treat every feature as an experiment that might be obsolete next quarter. That mindset shift drives three practices:
- Thin MVP slices: ship workflow value in days, not quarters.
- Model‑layer abstraction: swapping Gemma for Claude becomes a config change.
- Regular “sunset reviews”: each quarter PMs audit features built on older model assumptions and retire or refactor ruthlessly.
6.2 The Rise of Agent Librarians and AI Evangelists
Glean’s internal analytics show product managers are the heaviest creators of agents and advanced prompts, but adoption depends on a small cadre of enthusiasts in every function:
- They prototype agents (“Pipeline Hygiene,” “Roadmap Insights”).
- Package them into shareable templates.
- Coach peers on prompt safety and best practices.
Successful customers formalise that role—Agent Librarian or AI Champion—giving it explicit time and recognition so grassroots innovation scales beyond the first ten power users.
6.3 Security & Compliance Move Upstream
Because the agent touches sensitive data and can act autonomously, security review can’t be a last‑minute gate. Box inserts security engineers into the pod from sprint zero; Cohere bakes policy wiring (PII masks, prompt sanitation) into its SDK so product teams can’t skip it.
6.4 New Talent Mix
Old SaaS org | Post‑agent org |
---|---|
UX designer, PM, FE dev, BE dev, QA | UX designer, Prompt/agent engineer, Retrieval engineer, Policy/guardrail engineer, Observability/telemetry lead |
Quarterly release notes | Continuous “what did the agent learn this week?” digest |
Skills in highest demand:
- Retrieval engineering—optimising hybrid indexes, ACL‑aware search.
- Policy scripting & red‑teaming—anticipating prompt‑injection and jailbreak vectors.
- Outcome analytics—mapping agent actions to dollars saved or revenue won.
6.5 Leadership Playbook
- Mandate an AI budget line. Treat experiments as R&D, not side projects.
- Set a 90‑day agent OKR—e.g., “Automate 30 % of invoice triage.”
- Reward deletion. Celebrate teams that retire manual steps or legacy code after a successful agent rollout.
- Track learning velocity. Metrics: time from model release → production; number of agent templates reused across teams.
“We’ll all manage a team of AI agents just like a CEO manages chiefs of staff.” — Arvind Jain
Organisations that embrace that mental model—assigning owners, metrics and continuous training—will compound productivity fastest.
7. Real-World Challenges of Deploying AI Agents
Box, Glean, and Cohere have collectively worked with some of the world’s most data-sensitive, process-heavy enterprises—and their CEOs are surprisingly aligned on one thing: deploying AI in the enterprise is not a technical showcase, it’s a system-level negotiation with risk, inertia, and messy infrastructure.
7.1 Security, Permissions, and Data Boundaries Are Non-Negotiable
If there’s one universal deal-breaker, this is it. Enterprises, especially in finance and healthcare, are not asking for “flexibility”—they’re demanding full control over where models run and what data flows through them.
- “You can’t just turn on an AI thing that looks at all my data… it might expose secrets people shouldn’t have access to—because of outdated permission settings from years ago.”
— Aaron Levie, Box - “A lot of enterprise data is private… you must respect governance and permissions at a fine-grained level—even internally.”
— Arvind Jain, Glean - “That’s why we deploy on-prem or inside private VPCs. Without that, we don’t even get into the conversation.”
— Aidan Gómez, Cohere
Takeaway: Your agent isn’t production-ready until its retrieval and output are both security-compliant by default.
7.2 The Organization Isn’t Ready—Even If the Tech Is
Founders love roadmaps. Operators live in calendars. And it turns out most teams simply don’t have the bandwidth to rethink how they work, mid-flight.
- “You’re on the treadmill. Ten tasks a day. You don’t stop to redesign your workflow with AI.”
— Arvind Jain, Glean - “Sometimes the customer’s vision outpaces the actual data or system maturity. They want the agent to automate the full workflow, but the architecture isn’t there yet.”
— Aaron Levie, Box
Takeaway: Shipping a feature isn’t enough—you need internal champions, enablement loops, and permission to rethink process itself.
7.3 Trust Is the Bottleneck—Not Just Accuracy
Models are impressive. But hallucinations, prompt injection, and “weird edge cases” still kill production confidence.
- “Prompt injection is real. You can’t just throw LLMs into sensitive systems without serious guardrails.”
— Arvind Jain, Glean - “We’re seeing hesitation about putting AI-written code into production. The human wasn’t in the loop—who takes responsibility?”
— Aaron Levie, Box
Takeaway: Hallucination isn’t just a model problem—it’s a product risk. Design explainability, fallbacks, and approval paths into the system.
7.4 Architecture Lock-in Is a Real Threat
The space is evolving too fast to pick winners for the next 3 years. But the moment you hardwire agents to a vendor, data store, or orchestration flow—you’re betting more than you realize.
- “Ironically, the industry is moving so fast that what you need is architectural optionality. Don’t assume the vendor you pick now is the one you’ll want next year.”
— Aaron Levie, Box
Takeaway: Layer your stack. Separate retrieval, routing, orchestration, and model layers—so you can keep up when the market shifts.
7.5 Enterprise Buyers Are Overwhelmed by Choice—and Underinformed
The buyer’s inbox is full of AI startups. The feature pages all look the same. But most don’t know what questions to ask, or which products will still be standing in two years.
- “There are thousands of startups pitching AI to CIOs. Nobody knows which ones will survive—or how to evaluate them properly.”
— Arvind Jain, Glean
Takeaway: Product is only half the GTM. The other half is clarity, trust, and reference customers that de-risk the deal.
7.6 Talent Gaps and Missing Playbooks
Even internal product teams are flying blind. They’re used to deterministic APIs, not probabilistic agents.
- “There’s still a lack of knowledge around how to build these systems well. Most folks have maybe two years of experience max.”
— Aidan Gómez, Cohere
Takeaway: Treat internal tooling as R&D. Build prompt libraries, red-teaming rituals, and give teams permission to throw out what no longer fits.
Final Thought
If you’re building or deploying AI agents in the enterprise, technical capability is the floor, not the ceiling. Success will go to those who ship security-first, de-risk implementation, and give their customers—and internal teams—a way to evolve, not just adopt.
8. The Future of AI Agents
As AI agents move from experimentation to infrastructure, three of the most active players in enterprise AI—Arvind Jain (Glean), Aaron Levie (Box), and Aidan Gómez (Cohere)—offer a clear, if varied, vision of where this is all going. Here’s how they see the agent-native world unfolding.
Vision 1: “Everyone will have a team of AI agents”
Arvind Jain, Glean
Glean’s long-term bet is deeply personal: every knowledge worker will command their own personalized AI team—not just assistants, but co-workers and coaches who know your context and evolve with you.
“Our vision is to build the best AI team around every individual—assistants, coworkers, coaches—that help you become a 10x’er.”
Jain imagines a future where this isn't a luxury for executives, but a baseline for everyone—from fresh graduates to ops staff. These agents don’t just execute tasks—they understand your patterns, anticipate your needs, and actively coach improvement over time.
Vision 2: “READ → REASON → ACT as the new compute primitive”
Aaron Levie, Box
Levie sees AI agents as the next computing abstraction, replacing "apps" with autonomous flows. But his take is pragmatic: agents won’t replace all software, but they will disrupt unstrategic, over-built, or highly repetitive tooling.
“The 80% of consumer AI problems are solved—what remains is the messy, high-value 20%… ‘Book the flight, file the expense’—we’re not there yet.”
He forecasts agent-native software for narrow tasks (e.g. invoice parsing, internal IT workflows) becoming standard, while core systems (HR, CRM) remain owned by specialized SaaS vendors:
“Nobody wants to be responsible for rebuilding their HR system with AI. You don’t want to get sued by the EU because your agent missed a PII flag.”
For Levie, agents are about strategic augmentation, not total reinvention.
Vision 3: “Applied agent layers built on model breakthroughs”
Aidan Gómez, Cohere
Cohere’s focus is on deep enterprise embedding—less about flashy assistants, more about AI that’s surgically integrated into vertical workflows (e.g. summarizing longitudinal health records, auto-drafting insurance RFPs).
Gómez argues the future isn’t about replacing humans but amplifying them by layering reliable agent interfaces atop the best models:
“You want the best models, yes—but what matters more is making them usable. Agents aren’t just demos—they’re delivery systems.”
He sees the agent stack as threefold:
- A foundation model layer (language, vision, planning).
- A reasoning layer with structured input/output logic.
- A deployment layer with enterprise trust guarantees.
Converging Themes
All three agree on a few critical points:
- Autonomy is coming—but controllable, explainable autonomy.
- Workflows are the unit of value, not apps or interfaces.
- AI agents won’t replace entire products; they’ll replace steps inside them.
- Security, reasoning quality, and orchestration layers will determine long-term winners.
Strategic Implications
If you're building for this future:
- Design agents as products, not just features.
- Focus on precision in enterprise workflows, not open-ended generality.
- Embrace a modular stack: retrieval, reasoning, routing, and execution should be independently swappable.
- Invest in agent UX: trust, visibility, and fallback flows will define enterprise adoption.
Cheat‑Sheet Sidebar
Acronym | Meaning | Why it matters |
---|---|---|
RAG | Retrieval‑Augmented Generation | Fetches proprietary data so the LLM stays fresh and secure. |
VPC | Virtual Private Cloud | Mandatory deployment mode for finance & health data. |
Continuation pre‑train | Extending a base model with private corpus | Matches GPT‑4 quality in narrow domains for ≈$5 M, says Cohere. |
Prompt injection | Malicious attempt to hijack model instructions | Guardrail layer must sanitise inputs; red‑team regularly. |
Call to Action
- Forward this memo to your Staff PM or Eng Lead.
- Ask: “Which three workflows should we run through the Read → Reason → Act playbook this quarter?”
- Comment on this post with your candidate list—I’ll provide some feedback.
Member discussion